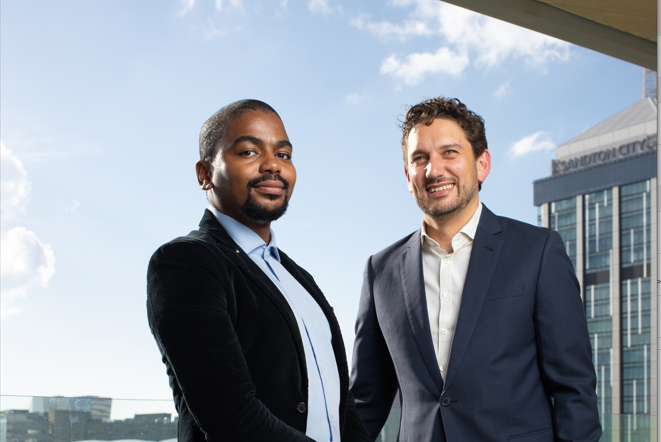
The media is awash with reports of technologies that are supposedly going to change the world, from continuous innovation in Web 3.0 and astonishing breakthroughs in artificial intelligence (AI) technologies to new ideas on monetary and fiscal policy (the so-called Modern Monetary Theory). And while many of these innovations might fizzle away, others will evolve, and some may indeed change the world as we know it. All we know is that it is a fool’s errand trying to forecast these trends and predict what the next technological frontier might hold. Our policy, therefore, is simply to remain adaptable, curious, and willing to embrace the new possibilities that these innovations could offer.
It is with this mindset that Mazi Asset Management embarked on a journey to explore the opportunities that AI and machine learning (ML) can offer both to us as an investment house and the investment community as a whole. Indeed, in March 2022, Mazi NextGen was launched with the sole focus of finding ways to incorporate ML techniques in investment practices.
Mazi NextGen emerged as a result of a collaboration between Stephán Engelbrecht, Alungile Gcaza and Mazi Asset Management. With a keen interest in ML, Stephán and Alungile had been delving into its various investment applications for some time, and eventually established a mutually beneficial partnership with Mazi.
The Mazi NextGen team has launched a long/short equity fund that will rely solely on ML techniques to identify and rank investment opportunities and construct the optimal portfolio from the resulting investment strategies. Moreover, the work of the Mazi NextGen team spans wider than just the new AI-driven hedge fund. Through extensive collaboration between the Mazi NextGen team and Mazi investment analysts, the whole firm’s ML strategies were improved, and enterprising ideas were developed on how ML techniques can be utilised in the broader Mazi investment process.
Drawing inspiration from concepts described by Bridgewater Associates’ Ray Dalio in his book Principles, we set out to create algorithms that leverage the collective investment principles of the Mazi investment team. These principles have been cultivated over decades of investment experience and are essential, in our view, to engendering shareholder trust and creating wealth over the long term. The incorporation of these fundamentals into Mazi’s ML algorithms is crucial, as the machines will consistently follow and prioritise the firm’s long-held investment principles and will be able to apply these to more data than humans could ever compute.
Below we discuss some of the areas where Mazi has been exploring the benefits of ML techniques in the broader investment process.
Hierarchical Clustering
At Mazi, we have come to learn that grouping companies superficially by the industry in which they operate is a dangerous way of constructing a portfolio.
As a simplistic example: although clothing retailers and food retailers may both be in the business of selling goods to consumers, their businesses are dependent on vastly different macro variables. For clothing retailers, macro variables, such as the credit cycle, GDP growth and savings rates, are critical to convincing consumers to spend their hard-earned money on discretionary items like clothing. Conversely, food retailers are far more sensitive to inflation and GDP per capita growth, as although consumers will always need to spend on food, it is the volume and value of these food items that will ultimately drive their gross profit margins. As such, clothing retailers may have much more in common with banks, while food retailers may share more likenesses with utilities than they do with one another.
Likewise, we always try to take a more exploratory look at the share price movements of the listed shares and then group them according to their statistical similarities, rather than superficial likenesses. We use the ML technique of “hierarchical clustering” to group shares. The groupings, as seen in Chart 1 below, make for some fascinating and logical insights, which are invaluable to Mazi portfolio managers in constructing portfolios with an improved risk profile.
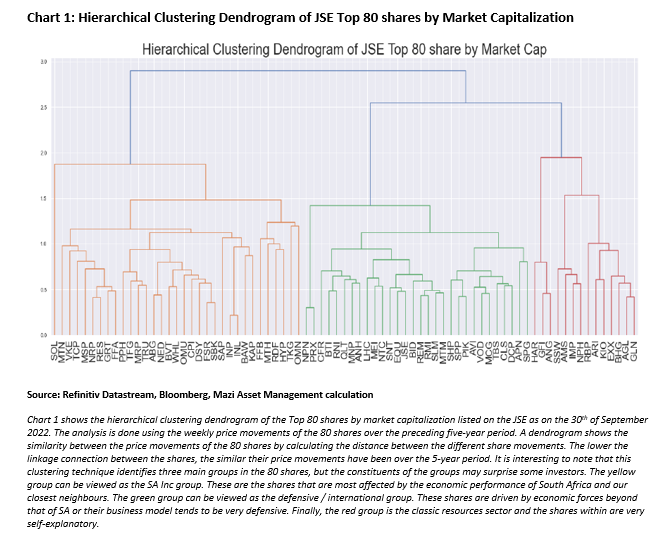
Principal Component Analysis and Clustering
In his memo to clients on 20 November 2001, titled You can't predict. You can Prepare., Oaktree Capital’s Howard Marks made the following statement:
"In my opinion, the key to dealing with the future lies in knowing where you are, even if you can't know precisely where you're going. Knowing where you are in a cycle and what that implies for the future is very different from predicting the timing, extent, and shape of the next cyclical move. And so, we'd better understand all we can about cycles and their behaviour."
At Mazi, we wholeheartedly subscribe to the notion that it is impossible to forecast economic events. We spend as little time as possible discussing macroeconomic events and even less trying to predict them. Instead, our approach is to focus on the bottom-up analysis of individual companies.
We are fully aware that economic events will have an impact on our investment performance and we therefore cannot be completely oblivious to the operating environment of companies. We must also be aware of which sectors, companies, and asset classes performed best or worst when considering historic periods when the macro environment most closely resembled the current environment. This will allow us to prepare for certain eventualities even if we cannot necessarily forecast them. This is also where ML capabilities will come into play.
Over the course of their long years of investment experience, our investment has identified approximately 28 factors that we consider to be the most relevant in describing the current economic and market landscape. These factors include, but are not limited to, local and international interest rates, market valuations, and market-, currency-, and commodity performances. Using principal component analysis and clustering algorithms, our algorithm can collate economic and market conditions and calculate the reasonable proximity of past and historic conditions to current market conditions. We can then use these timestamps to explore the performance of sectors, companies, and asset classes over a certain period.

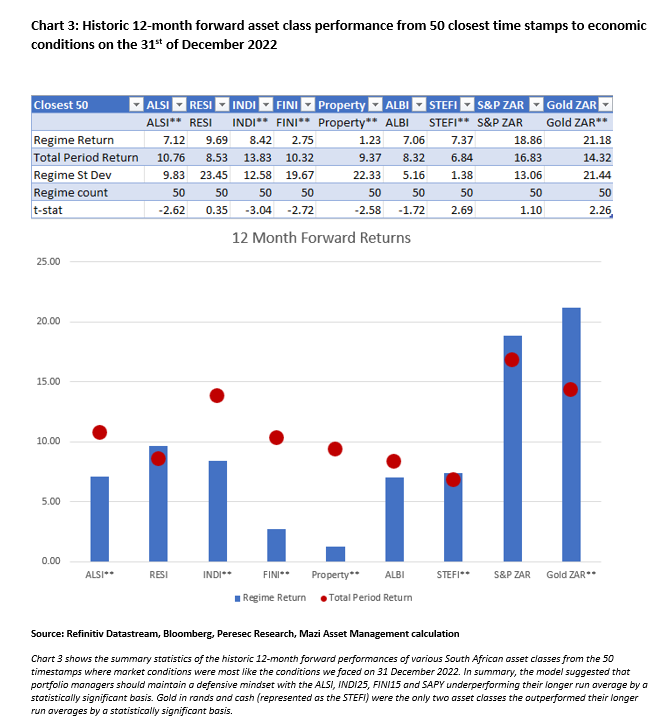
Idea Generation
In 1970, a seminal paper, Man versus Model of Man: A rationale, plus some evidence, for a method of improving on clinical inferences, was released by Professor Lewis Goldberg from the University of Oregon. In his paper, Professor Goldberg showed that computer models built from the knowledge and experience of clinical psychologists were more consistent and more reliable than humans (whose knowledge and experience were used in developing the models) to accurately diagnose new patients. The reasoning behind this is simple: humans have exceptional knowledge and experience, but they are prone to place too much emphasis on certain variables –perhaps with recency bias for those variables that they may have been studying recently – or be too optimistic or too pessimistic on any given diagnosis. Machines do not suffer these biases or emotions.
We believe that a similar case can be made for investment management. As such, we have developed ML algorithms that utilise the fundamental, momentum and technical variables that we believe are important when analysing companies. These algorithms have the capability to analyse our entire investment universe and identify potential investment opportunities that we humans may have missed.
It is important to note that these are not simple linear screening models. Indeed, these ML algorithms are non-linear and multi-layered. In statistical terms, this means the model will consider conditionalities when calculating the probability of success. In layman’s terms and to use a simplistic example, the algorithm will for instance identify that a company exhibiting low earnings volatility and reasonable price momentum that historically generated a 20% return on equity (ROE) and is currently trading on a 10x Price-to-Earnings multiple is more attractive than a company with low or negative price momentum that was only able to generate an ROE of 8% trading on the same 10x Price-to-Earnings multiple. The algorithms will identify these conditionalities by building hundreds of classification trees that group companies as outperformers and underperformers given certain characteristics.
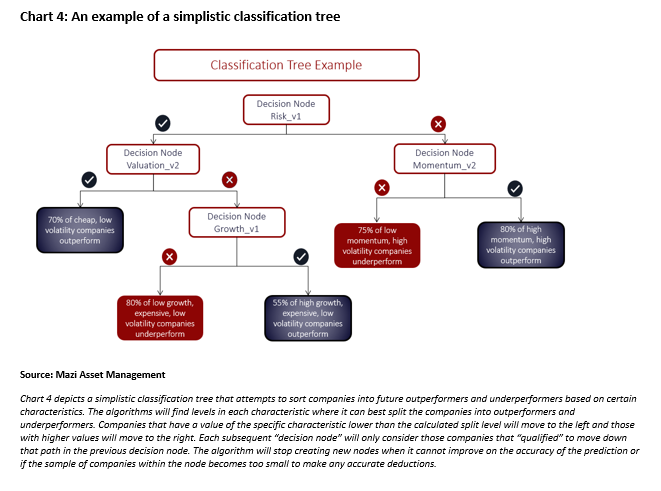
These models can often identify opportunities that we may have missed, provide us with the confidence needed to follow through on a potentially contrarian view that we may have identified, or, conversely, question our logic for holding a company that has a low probability of success. In general, our ML model offers an independent, and quantitatively-rooted view of our investment portfolio that keeps us alert, agile, and open to new ideas and opportunities.
The above are just three of the many projects that we are currently exploring, and we believe we are still very much on tip-of-the-iceberg territory, which is why we are determined to remain at the forefront of the developments in ML and AI to continually improve our investment process.
At Mazi, we are always looking to improve and fine-tune our processes, and the AI revolution may be an exciting step forward for our team and clients.
We would like to assure our investors that we are at the very forefront of ML technological exploration and we welcome them to come along for the ride.